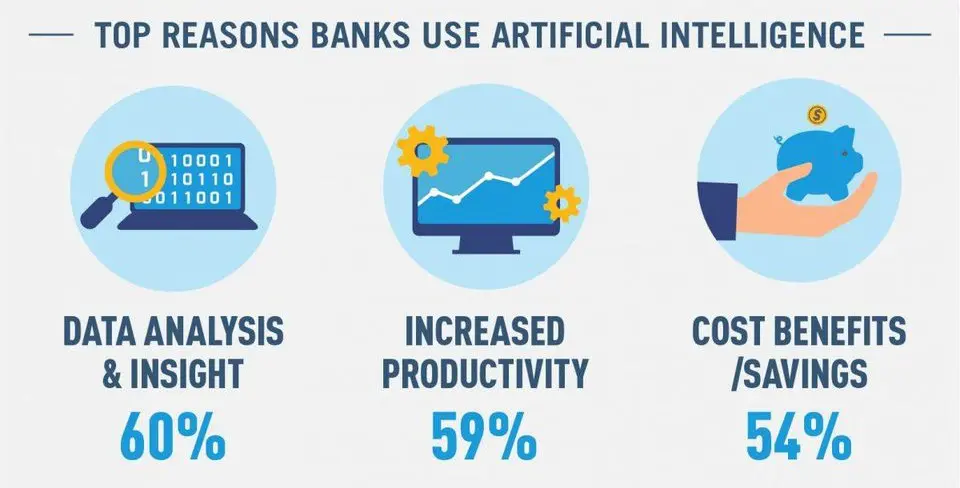
How Banks Can Use Artificial Intelligence (AI) and Machine Learning (ML) In Their Operations
Banks are pretty old-school when it comes to their digital banking solutions, but times are changing, and banks need to keep up. The banking industry is undergoing a major transformation, and it’s not just about the financial institutions’ physical appearance.
The landscape is changing, and banks are scrambling to adapt to the new reality. Online banking has become more popular, and many people are opting to do their banking from the comfort of their homes.
Banks have started to embrace artificial intelligence and machine learning for various reasons. The rising awareness of the dangers of human error, the need to create a more personalized experience, and
Ways in Which AI and ML Will Shape the Banking and Finance Sector Front-Office Use Cases of AI and ML in Banking
- Conversational Chatbots and Virtual Assistants
Bank customers seek a more conversational experience. Customer service like that offered by Amazon, Netflix, and Uber is what they desire. In banking, chat bots and virtual assistants make this feasible because of their advancement.peopletools att
They demand the same degree of customer care from their banks as they do for the rest of their lives. If you have a question about your bank account or other transactions, chatbots and virtual assistants can help you 24/7. Customers may also use natural language to transmit money.
- Customer Sentiment Analysis
Big data and machine learning are increasingly being used by banks to better understand their customers’ emotional states. There is a plethora of information about their clients that banks currently hold, but most of it is unstructured and hence difficult for computers to grasp. On the other hand, Artificial intelligence is capable of making sense of this data and deriving new insights from it.
Customer service agents might use artificial intelligence to read the customer’s emotions when they speak to the help desk. Customers’ problems may be alleviated by determining whether or not banks’ employees are handling issues successfully.
- ML-Driven Underwriting Processes
It is difficult to evaluate a customer’s creditworthiness if they have no prior credit history with the bank. Big data and machine learning examine more than 10,000 data points to determine a person’s creditworthiness. Thus, pre-approved loans may be given to a wide variety of consumers, including students and self-employed people.
Artificial intelligence-based underwriting may simplify even the most complicated processes in corporate lending, evaluate market trends, detect loan risks, predict future behavior, assess the possibility of fraud, and so on.
- Personalized Banking
Artificial intelligence is still in its infancy as a technology in the banking industry. Predictive analytics, for example, is already being used by certain banks to identify and anticipate consumers’ financial requirements.
Banks must first learn how their customers prefer to be addressed to truly personalize banking. This is just the beginning. Much work is required here. Banks can’t keep track of all the information they need since it’s scattered across several systems and departments. With AI, this information may be mined and sent to the consumer with relevant insights or suggestions.
Back Office Use Cases of AI and ML in Banking
- Intelligent Automation
Many manual operations will be automated by ML solutions using intelligent process automation and Oracle digital banking experience, freeing workers from having to do such jobs by hand. It is feasible to automate processes in the banking industry by using chatbots, staff training gamification, and so on. Banks can increase their operations and save on expenses simultaneously by using this technology.
- Integrated Command and Control Systems
Nowadays, banks and other financial organizations are required to handle personal information about their own customers and those of third parties. As a result, data is stored in separate silos, making it impossible to have one central repository with all relevant information. As a result, banks cannot make better judgments since they cannot examine all their data.
Data engineering and machine learning methods may be used to merge the many data sources into a single source of truth. An acronym for this would be “Integrated Command and Control Center.” Banks can now make better judgments based on their accessible data rather than just a part of it.
Trading and Portfolio Management Use Cases of AI and ML in Banking
- Wealth and Portfolio Management
The AI-based algorithms can even determine who the company’s prospective investors are based on their income and spending habits. It can also evaluate market trends and select suitable funds depending on the portfolio. Because this can be done remotely, there is no need to ever set foot in your branch to complete it.
- Algorithmic Trading
A machine learning-based trading method is a certainty. If you’re looking for the best of both worlds, you may consider using an algorithmic trading platform, which uses computers to execute trades instead of humans.
While algorithmic trading is well-known, we are also seeing an increase in machine-learning algorithms in banking. Machine learning algorithms can work on a vast scale without the assistance of a data scientist. Their ability to analyze vast amounts of data at once enables them to derive more accurate judgments than any individual trader.
Regulatory Compliance (RegTech) and Supervision (SupTech) Use Cases of AI and ML in Banking
- Cybersecurity and Anti-Money Laundering
Soon, banking will become safer thanks to the creative use of AI and machine learning in the financial sector. The financial services sector will benefit from applying AI and ML to improve their cybersecurity and anti-money laundering activities.
The use of AI and machine learning technology by banks is on the rise to spot any transactions that could be indicative of money laundering operations. Artificial intelligence and machine learning are projected to see tremendous growth.
Incorporating these technologies will result in a more efficient service, saving money and time. As a result, banks, organizations, and people will be able to better protect their institutions, networks, and accounts against cyberattacks.
- AI-Powered Fraud Detection
ML algorithms can sift through billions of data points to find evidence of fraud. Approvals are more accurate in real-time, with fewer incorrect rejections. Rather than waiting for the crime to occur before identifying suspicious activity, it will do it in real-time.
At least $2.92 is spent by financial institutions to recoup every dollar they lose to fraud. These technologies have the potential to transform the banking industry. Monitoring methods used by banks are often based on past payment information. Machine learning-powered algorithms can readily distinguish between fraudulent and legitimate credit card transactions since they are built on a massive database of transaction data.
Conclusion
In banking, AI and machine learning are currently at a very early stage. Still, there is reason to be optimistic about their future growth. It’s good that financial institutions are starting to grasp the importance of technologies like OBDX. When it comes to becoming a technology-powered business, most banks have inflexible procedures that provide substantial operational and organizational hurdles. Trust in these technologies and preparation for adoption are necessary to overcome this challenge.